
- This event has passed.
16/12/2020 – AI3SD Winter Seminar Series: Enhancing Experiments through Machine Learning
16th December 2020 @ 2:00 pm - 4:45 pm
Free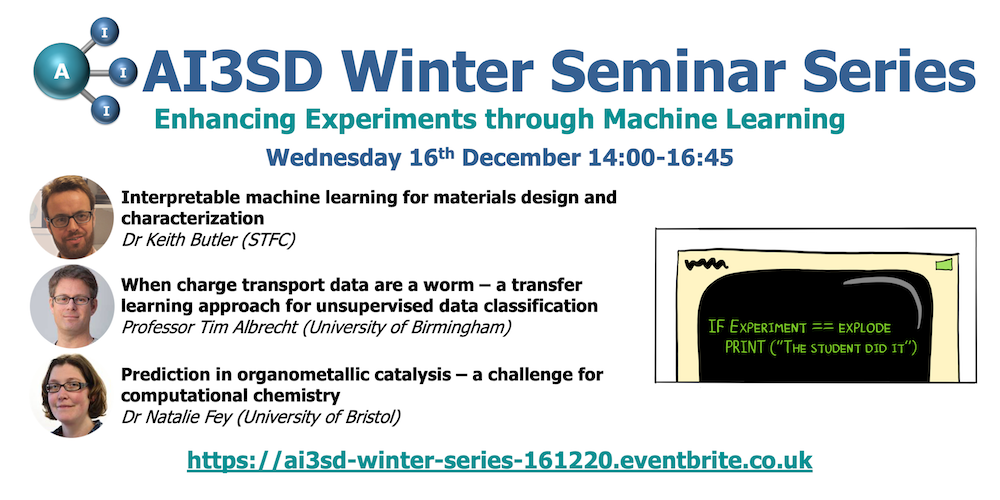
Eventbrite Link: https://ai3sd-winter-series-161220.eventbrite.co.uk
Description:
This seminar forms part of the AI3SD Online Seminar Series that will run across the winter (from November 2020 to April 2021). This seminar will be run via zoom, when you register on Eventbrite you will receive a zoom registration email alongside your standard Eventbrite registration email. Where speakers have given permission to be recorded, their talks will be made available on our AI3SD YouTube Channel. The theme for this seminar is Enhancing Experiments through Machine Learning.
Agenda
- 14:00-14:45: Interpretable machine learning for materials design and characterization – Dr Keith Butler (STFC)
- 14:45-15:00: Coffee Break
- 15:00-15:45: When charge transport data are a worm – a transfer learning approach for unsupervised data classification – Professor Tim Albrecht (University of Birmingham)
- 15:45-16:00: Coffee Break
- 16:00-16:45: Prediction in organometallic catalysis – a challenge for computational chemistry – Dr Natalie Fey (University of Bristol)
Abstracts & Speaker Bios
Interpretable machine learning for materials design and characterization – Dr Keith Butler
Abstract: In a plenary lecture at a recent international conference, one leading researcher in theoretical chemistry remarked “at least 50% of the machine learning papers I see regarding electronic structure theory are junk, and do not meet the minimal standards of scientific publication”, specifically referring to the lack of insight in many publications applying ML in that field. But is knowledge inevitably lost in machine learning studies, if not how can it be extracted and how does this apply to machine learning in the context of materials science? In this talk I will look at how we can open up black box machine learning models, to understand the results and gain confidence in predictions. I will present topical examples from designing new dielectric crystals, understanding inelastic neutron scattering data and trusting deep neural networks for tomographic reconstruction. By understanding how and why these models work, we can trust the results and even discover new physical relationships.
Bio: Keith Butler is as a senior data scientist working on materials science research in the SciML team at Rutherford Appleton Laboratory. SciML is a team in the Scientific Computing Division and we work with the large STFC facilities (Diamond, ISIS Neutron and Muon Source and Central Laser Facility for example) to use machine learning to push the boundaries of fundamental science.
When charge transport data are a worm – a transfer learning approach for unsupervised data classification – Professor Tim Albrecht
Abstract: Advanced data analysis methodologies, and in particular dimensionality reduction techniques, are now used more and more widely in the single-molecule charge transport community. They allow for comprehensive exploration of large datasets, where data display significant variance and sometimes contain (unknown) sub-populations. To this end, unsupervised approaches, which do not rely on class labels or pre-defined expectations can be advantageous. Multi-Parameter Vector Classification (MPVC) is one example and PCA-based methods have also been employed in this context [1,2,3]. We have recently shown how Transfer Learning may be employed to identify and quantify hidden features in single-molecule charge transport data [3]. Using open-access neural networks such as AlexNet, trained on millions of seemingly unrelated image data, feature recognition then does not require network training with application-specific data. Instead, the network recognises features in the input that it had learned in other contexts and, for example, identifies different shapes in conductance-distance traces as images of different worm species. Thus, our results show how Deep Learning methodologies can readily be employed for unsupervised data classification, even if the amount of problem-specific, ‘own’ data is limited.
[1] M Lemmer, MS Inkpen, K Kornysheva, NJ Long, T Albrecht, “Unsupervised vector-based classification of single-molecule charge transport data”, Nat. Comm. 2016, 7, 12922.
[2] T Albrecht, G Slabaugh, E Alonso, SMMR Al-Arif, “Deep learning for single-molecule science”, Nanotechnology 2017, 28 (42), 423001.
[3] A Vladyka, T Albrecht, “Unsupervised classification of single-molecule data with autoencoders and transfer learning”, Mach. Learn.: Sci. Technol. 2020, 1, 035013.
Bio: Tim studied Chemistry at the University of Essen in Germany from 1995-2000. Following brief research visits at the European Joint Research Centre in Ispra in Italy and the University of California at Berkeley, Tim graduated with a Diploma in Chemistry (equivalent to a Masters degree) in early 2000. After graduating, Tim joined Peter Hildebrandt’s group at the Max-Planck Institute for Radiation Chemistry (now Bioinorganic Chemistry) in 2000. Tim worked on charge transfer processes in natural and artificial heme proteins on metal surfaces using SER(R)S, single-crystal electrochemistry and electrochemical STM (in Jens Ulstrup’s group at the Technical Institute of Denmark (DTU). He obtained his PhD from the Technical University (TU) of Berlin in 2003 and afterwards returned to Ulstrup’s group as a postdoctoral fellow. In 2006, he moved to London to take up a lecturer position in Interfacial and Analytical Sciences in the Chemistry Department at Imperial College, where he was made Senior Lecturer in 2011 and then Reader in 2014. In 2017, Tim joined the faculty in the School of Chemistry at Birmingham University as Chair of Physical Chemistry and has been the School of Chemistry’s Director of Research since 2018. He is the coordinator of the School’s Interest Group “Data and Machine Intelligence”.
Prediction in organometallic catalysis – a challenge for computational chemistry – Dr Natalie Fey
Abstract: Computational results are now routinely used to contribute to the interpretation of experimental data, including for the confirmation of mechanistic postulates, but their contribution to substantial predictions made before experiments remains the exception [1], at least in the area of organometallic catalysis. More effective use of what we know about chemical reactions, regardless of whether the information was generated from experiment or calculation, will clearly play a role in moving towards this kind of ab initio prediction in this field. Here the adoption of statistics and data science into the chemical sciences are proving crucial and we have built large databases of parameters characterising ligand and complex properties in a range of different environments [2-6]. In this session, I will use examples drawn from our recent work, including the early stages of our development of a reactivity database, to illustrate this approach and discuss why organometallic catalysis is such a challenging yet rewarding area for prediction.
Website: https://feygroupchem.wordpress.com/
References:
1. J. Jover, N. Fey, Chem. Asian J., 9 (2014), 1714-1723; D. J. Durand, N. Fey, Chem. Rev., 119 (2019), 6561-6594.
2. A. Lai, J. Clifton, P. L. Diaconescu, N. Fey, Chem. Commun., 55 (2019), 7021-7024.
3. O. J. S. Pickup, I. Khazal, E. J. Smith, A. C. Whitwood, J. M. Lynam, K. Bolaky, T. C.
King, B. W. Rawe, N. Fey, Organometallics, 33 (2014), 1751-1791.
4. J. Jover, N. Fey, J. N. Harvey, G. C. Lloyd-Jones, A. G. Orpen, G. J. J. Owen-Smith, P.
Murray, D. R. J. Hose, R. Osborne, M. Purdie, Organometallics, 29 (2010), 6245-6258.
5. J. Jover, N. Fey, J. N. Harvey, G. C. Lloyd-Jones, A. G. Orpen, G. J. J. Owen-Smith, P.
Murray, D. R. J. Hose, R. Osborne, M. Purdie, Organometallics, 31 (2012), 5302-5306.
6. A. I. Green, C. P. Tinworth, S. Warriner, A. Nelson, N. Fey, Chem. Eur. J. 2020, Accepted Article, DOI: 10.1002/chem.202003801.
Bio: I was born in Frechen, Germany, but have lived and worked in the UK for quite a while now. I obtained my BSc in Chemistry and Economics from Keele University (UK), and stayed on to work with Jim Howell and Paul Yates towards a PhD (completed in 2001). After postdoctoral research with Rob Deeth at the University of Warwick until 2003, I worked as a postdoc on projects with Guy Orpen, Jeremy Harvey and Guy Lloyd-Jones at the University of Bristol before gaining an EPSRC Advanced Research Fellowship (October 2007). My independent research at Bristol is in computational inorganic chemistry and involves mechanistic studies of catalysis and the development of knowledge bases. I was appointed to a temporary lectureship in 2015, made permanent in 2017, and promoted to senior lecturer in 2018. I’m the programme director for Chemistry with Scientific Computing and the Deputy Director of Bristol Scientific Computing.