All aspects of the Network will be developed through co-creation from amongst the members. Experience in running networks has shown us that finding the right language and approach to communication is essential for true co-creative and meaningful collaborations. For the proposed network this will be facilitated by making use of the philosophical underpinnings of AI & Discovery to provide a common language that enables the AI and Physical Science communities to engage in joint endeavours. The output of these endeavours will impact directly on the nature of what discovery & theory will mean in the next decades and on the skills set that the AI generation of researchers will need to develop.
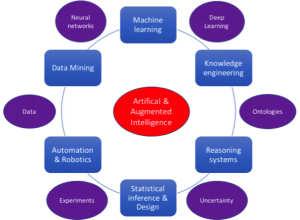
Areas of Chemical, Physical and Material Sciences
- Chemicals and Materials Discovery: We will be pushing the limits of synthesis, properties and engineering of molecules and materials, enabling the discovery and exploitation of unseen and un-imagined parts of chemical and material space.
Areas of AI
- Types of AI: We will investigate the impact of different types of AI modalities (e.g. pattern recognition vs reasoning AI, narrow vs broad AI) on scientific discovery. We will also explore what limits might exist in probing machine learning (AI) models to derive the rationale and reasons for the outputs of the models. This will enable us to map AI properties or transient states onto the more traditional approach to theory. As machine learning moves from addressing small scale text-book problems in statistical pattern recognition to being parts of deployable systems that can be integrated into discovery science roles, two important challenges, Privacy and Provenance, need to be addressed. (a) Privacy preserving learning, whereby the owners of data from domains in which privacy matters may be unwilling to share data. In such scenarios, algorithmic advances that can work with minimal statistical information about data, rather than working with raw data, will need to be developed (b) Provenance: Learning systems that are embedded in a discovery setting will need to be cognisant of the provenance of data used for their training. As multiple sources of data become available, particularly when experimental data are archived in public repositories, uncertainty about them is bound to increase. The frontiers of machine learning need to be pushed to cope with varying reliability of the available data.
- Agents & Swarms: How much can AI for Scientific Discovery make use of, and learn, from the expert game players? We will push the frontiers of Deep Reinforcement Learning whereby agent-based modelling at a “high level” can take advantage of data-driven models at a lower level. These two topics have developed largely separately in the literature, and bringing them together is crucial for discovery science in that reinforcement learning is suitable for planning and experiment design whereas data-driven modelling is needed to extract useful information from large and complex experimental data. Inducing strong coupling between the two is an open-ended challenge that needs to be addressed.
- Evolutionary approaches & Understanding the AI results: Conventional applications of AI tend to work in well-defined problem domains (clear objective functions, supervised learning methods, data-driven deduction) but scientific discovery is often ad hoc and opportunistic, with discoveries that can arise in unexpected ways and in ‘problems’ that are ill-defined at the outset. The human capability for discovery in such domains is surpassed only by natural evolutionary processes. Operating in a purely non-teleological way, biological evolution is the epitome of such an ad hoc and opportunistic process. Recent breakthroughs in two specific areas provide an opportunity to exploit artificial evolutionary processes for scientific discovery in a new way: (a) ‘distilling natural laws from experimental data’; (b) Exploiting the formal equivalence of natural evolution and machine learning. In general, what is the impact of the understanding the results and therefore the ethical basis for using the resulting models.