
- This event has passed.
01/12/2021 – AI3SD Autumn Seminar VIII: Molecules, Graphs & Networks
1st December 2021 @ 2:00 pm - 3:45 pm
Free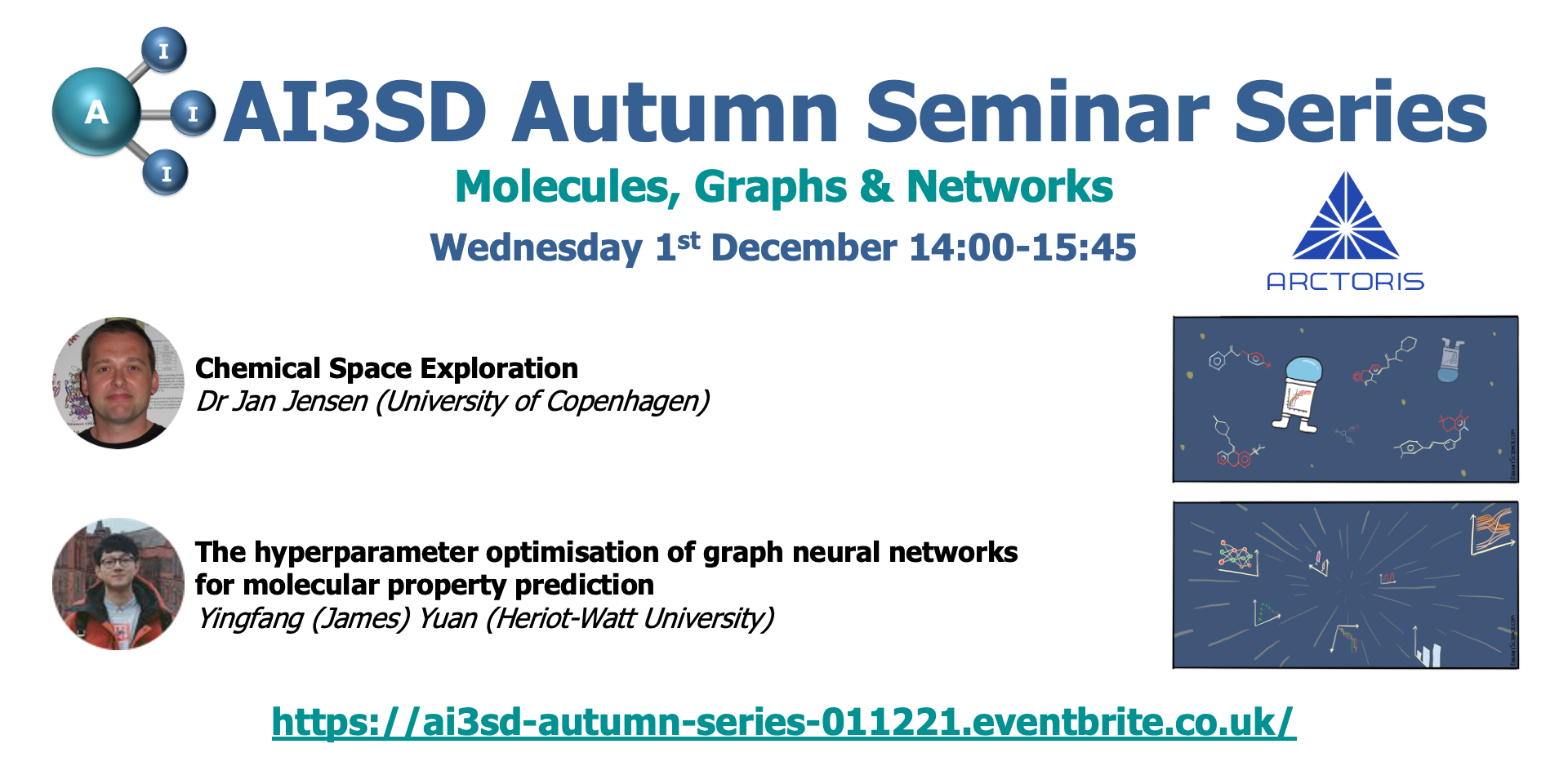
Eventbrite Link: https://ai3sd-autumn-series-011221.eventbrite.co.uk
Description:
This seminar forms part of the AI3SD Online Seminar Series that will run across the autumn (from October 2021 to December 2021). This seminar will be run via zoom, when you register on Eventbrite you will receive a zoom registration email alongside your standard Eventbrite registration email. Where speakers have given permission to be recorded, their talks will be made available on our AI3SD YouTube Channel. The theme for this seminar is Molecules, Graphs & Networks.
Agenda
- 14:00-14:45: Chemical Space Exploration – Professor Jan Jensen (University of Copenhagen)
- 14:45-15:00: Coffee Break
- 15:00-15:45: The hyperparameter optimisation of graph neural networks for molecular property prediction – James Yuan (Heriot Watt University)
Abstracts & Speaker Bios
- Chemical Space Exploration – Jan Jensen: We explain why genetic algorithms can find molecules with particular properties in an enormous chemical space (ca 10^60 molecules) by considering only a tiny subset(typically 10^3−6 molecules). I show how genetic algorithms can be used to optimise optical, binding, and catalytic properties of molecules, while ensuring synthetic accessibility.
Bio: Jan H. Jensen obtained his PhD in theoretical chemistry in 1995 from Iowa State University working with Mark Gordon, where he continued as a postdoc until he joined the faculty at theUniversity of Iowa in 1997. In 2006 he moved to the University of Copenhagen, where he is now professor of computational chemistry. - Hyperparameter Optimisation for Graph Neural Networks – James Yuan: Traditional deep learning has made significant progress on various problems, from computer vision to natural language processing. For graph problems, there are still many challenges. Graph neural networks (GNNs) have been proposed for a wide range of learning tasks in the graph domain. In particular, in recent years, an increasing number of GNN models were applied to model molecular graphs and predict the properties of the corresponding molecules. However, a direct impediment to achieve good performance with the lower computational cost is to select appropriate hyperparameters. Meanwhile, many molecular datasets are far smaller than many other datasets in typical deep learning applications. Most hyperparameter optimization (HPO) methods for deep learning have not been explored in terms of their efficiencies on such small datasets in the molecular domain. We conducted theoretical analyses for popular HPO methods (random search, TPE, and CMA-ES) and proposed a genetic algorithm with hierarchical evaluation strategy and tree-structured mutation for HPO. Finally, we believe that our work will motivate further research to GNNs as applied to molecular machine learning problems and facilitate scientific discovery.
Bio: Yingfang (James) Yuan is a PhD candidate working under the supervision of Dr Wei Pang, Prof. Mike Chantler and Prof. George M. Coghill (external) in the School of Mathematical and Computer Sciences at Heriot-Watt University. Yingfang received his MSc in Big Data and High-Performance Computing at University of Liverpool. His research interests include Machine Learning; Deep Learning (especially in Graph Neural Networks and AutoML); He particularly focused on investigating the impact of hyperparameter optimization on graph neural networks applied to predict molecular properties and the efficiency of hyperparameter optimization approaches.