
- This event has passed.
24/02/2021 – AI3SD Winter Seminar Series: Materials Machine Learning (MML)
24th February 2021 @ 2:00 pm - 4:45 pm
Free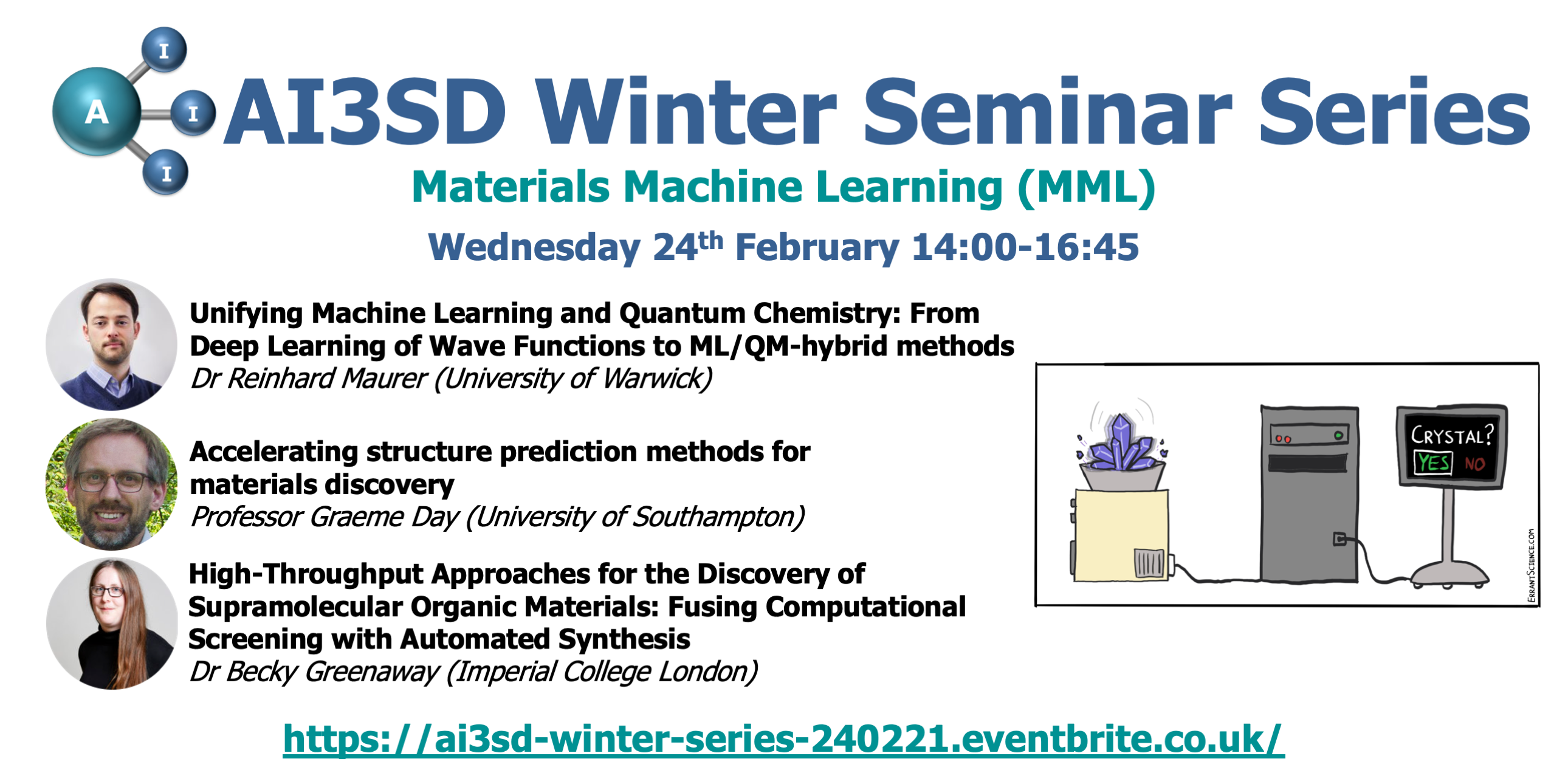
Eventbrite Link: https://ai3sd-winter-series-240221.eventbrite.co.uk
Description:
This seminar forms part of the AI3SD Online Seminar Series that will run across the winter (from November 2020 to April 2021). This seminar will be run via zoom, when you register on Eventbrite you will receive a zoom registration email alongside your standard Eventbrite registration email. Where speakers have given permission to be recorded, their talks will be made available on our AI3SD YouTube Channel. The theme for this seminar is Materials Machine Learning (MML).
Agenda
- 14:00-14:45: Unifying Machine Learning and Quantum Chemistry: From Deep Learning of Wave Functions to ML/QM-hybrid methods – Dr Reinhard Maurer (University of Warwick)
- 14:45-15:00: Coffee Break
- 15:00-15:45: Accelerating structure prediction methods for materials discovery: Professor Graeme Day (University of Southampton)
- 15:45-16:00: Coffee Break
- 16:00-16:45: High-Throughput Approaches for the Discovery of Supramolecular Organic Materials: Fusing Computational Screening with Automated Synthesis – Dr Becky Greenaway (Imperial College London)
Abstracts & Speaker Bios
- Deep Learning Enhanced Quantum Chemistry: Pushing the limits of Materials Discovery – Dr Reinhard Maurer: Atomistic simulation based on quantum mechanics (QM) is currently being revolutionized by machine-learning (ML) methods. Many existing approaches use ML to predict molecular properties from quantum chemical calculations. This has enabled molecular property prediction within vast chemical compound spaces and the high-dimensional parametrization of energy landscapes for the efficient molecular simulation of measurable observables. However, as all properties derive from the QM wave function, an ML model that is able to predict the wave function also has the potential to predict all other molecular properties. In this talk, I will explore ML approaches that directly represent wave functions and QM Hamiltonians and their derivatives for developing methods that use ML and QM in synergy. [1] Using examples from molecular dynamics [1] and heterogeneous catalysis, [2,3] I will discuss the challenges associated with encoding physical symmetries and invariance properties into deep learning models. Upon overcoming these challenges, integrated ML-QM methods offer the combined benefits of big-data-driven parametrization and first-principles-based methods. I will discuss several opportunities associated with building ML-augmented quantum chemical methods, including Inverse Chemical Design based on ML-predicted wave functions and the development of efficient and accurate semi-empirical methods to study hybrid metal-organic materials. [4]
[1] KT Schütt, M Gastegger, A Tkatchenko, K-R Müller & RJ Maurer, Nature Communications 10, 5024 (2019).
[2] Y Zhang, RJ Maurer, and B Jiang, J. Phys. Chem. C 124, 186-195 (2020);
[3] Y Zhang, RJ Maurer, H Guo, and B Jiang, Chem. Sci. 10, 1089-1097 (2019).
[4] M Gastegger, A McSloy, M Luya, KT Schütt, RJ Maurer, J. Chem. Phys. 153, 044123 (2020).
Bio: Reinhards research focuses on the theory and simulation of molecular reactions on surfaces and in materials. Reinhard studies the structure, composition, and reactivity of molecules interacting with solid surfaces. Reinhards goal is to find a detailed understanding of the explicit molecular-level dynamics of molecular reactions as they appear in catalysis, photochemistry, and nanotechnology. Members of Reinhards research group develop and use electronic structure theory, quantum chemistry, molecular dynamics, and machine learning methods to achieve this. - Accelerating structure prediction models for materials discovery – Professor Graeme Day: The discovery of new functional materials can be guided by computational screening, particularly if the structure of a material can be reliably predicted from its chemical composition. For this application, we have been developing the use energy-structure-function maps [1], which summarise the crystal structures available to a given molecule and the relevant properties that are predicted for these structures. The use of these methods is still limited by the computational cost of crystal structure prediction (CSP). Most of the cost of CSP is associated with the calculation of the relative energies of predicted crystal structures using energy models that are sufficiently accurate to provide reliable energetic rankings. To speed up these methods, we have been developing machine learning approaches to predict high quality energies (e.g. from solid state density functional theory) from structures that have been generated with computationally efficient energy models [2-4]. The talk will discuss the performance of these methods, which use Gaussian Process Regression based on descriptors of local environments of atoms within crystal structures. I will also describe how these descriptors can be used to more quickly navigate the structure-property landscapes of molecular crystals [5] and how fast CSP can be applied to screen chemical space for the most promising molecules for a given application [6].
[1] Functional materials discovery using energy–structure–function maps, A. Pulido et al, Nature 2017, 543, 657.
[2] Machine learning for the structure–energy–property landscapes of molecular crystals, F. Musil, S. De, J. Yang, J. E. Campbell, G. M. Day and M Ceriotti, Chem. Sci. 2018, 9, 1289-1300.
[3] Machine-Learned Fragment-Based Energies for Crystal Structure Prediction, D. McDonagh, C.-K. Skylaris and G. M. Day, J. Chem. Theory Comput. 2019, 15, 2743–2758
[4] Multi-fidelity Statistical Machine Learning for Molecular Crystal Structure Prediction, O. Egorova, R. Hafizi, D. C. Woods and G. M. Day, J. Phys. Chem. A 2020, 124, 39, 8065–8078.
[5] Distributed Multi-Objective Bayesian Optimization for the Intelligent Navigation of Energy Structure Function Maps For Efficient Property Discovery, E. Pyzer-Knapp, G. M. Day, L. Chen, A. I. Cooper, ChemRxiv 2020, https://doi.org/10.26434/chemrxiv.13019960.v1
[6] Evolutionary chemical space exploration for functional materials: computational organic semiconductor discovery, C. Y. Cheng, J. E. Campbell and G. M. Day, Chem. Sci. 2020, 11, 4922-4933.
Bio: Graeme Day is Professor of Chemical Modelling at the University of Southampton. His research concerns the development of computational methods for modelling the organic molecular solid state. A key focus of this work is the prediction of crystal structures from first principles; his research group applies these methods in a range of applications, including pharmaceutical solid form screening, NMR crystallography and computer-guided discovery of functional materials. After a PhD in computational chemistry at University College London, he spent 10 years at the University of Cambridge, where he held a Royal Society University Research Fellowship working mainly on modelling pharmaceutical materials and computational interpretation of terahertz spectroscopy. He moved to the University of Southampton in 2012, at which time he was awarded a European Research Council Starting Grant for the ‘Accelerated design and discovery of novel molecular materials via global lattice energy minimisation’ (ANGLE). This grant shifted the focus of his research to functional materials, including porous crystals and organic electronics. In 2020, he was awarded an ERC Synergy grant ‘Autonomous Discovery of Advanced Materials’ (ADAM) with Andrew Cooper (Liverpool) and Kerstin Thurow (Rostock) to integrate computational predictions, chemical space exploration with automation in the materials discovery lab. Graeme has served on the editorial boards of CrystEngComm, Faraday Discussions and on the advisory board of Molecular Systems Design & Engineering (MSDE). - High-Throughput Approaches for the Discovery of Supramolecular Organic Materials: Fusing Computational Screening with Automated Synthesis’? – Dr Becky Greenaway: Dynamic covalent chemistry is a powerful tool for assembling complex organic molecules, such as macrocycles, cages, and catenanes. These supramolecular organic molecules can be synthesised using a range of enabling technologies, including flow chemistry,[1] mechanochemistry,[2] and high-throughput automation,[3] enabling both their scale-up and the ability to screen a broad synthetic space. However, when applied blindly, high-throughput automation is inefficient, and the targeted design of such molecules can be challenging, especially as the systems become more elaborate, because multi-component assembly reactions have the potential to form a wide range of different species. In an attempt to address this, in collaboration with the Jelfs group, we have been developing hybrid workflows that fuse computational screening with robotic synthesis for the discovery of new supramolecular organic materials.[3-5] In this talk, I will give an overview of some of the different hybrid workflows that have been used in the discovery new organic cages, covalently-bridged catenanes, socially self-sorted multi-component cage pots, and organic cage dumbbells, giving an overview of how we are trying to move away from a posteriori rationalisation towards a priori prediction. I will also discuss several opportunities for the incorporation of machine-learning[6] and evolutionary algorithms[7] into these workflows, with the overall aim of designing new supramolecular organic materials in a closed feedback loop.
[1] Dynamic flow synthesis of porous organic cages, M. E. Briggs et al, Chem. Commun., 2015, 51, 17390
[2] Continuous and scalable synthesis of a porous organic cage by twin screw extrusion (TSE), B. D. Egleston et al, Chem. Sci., 2020, 11, 6582
[3] High-throughput discovery of organic cages and catenanes using computational screening fused with robotic synthesis, R. L . Greenaway et al, Nature Commun., 2018, 9, 2849
[4] Computationally-inspired discovery of an unsymmetrical porous organic cage, E. Berardo et al, Nanoscale, 2018, 10, 22381
[5] From concept to crystals via prediction: multi-component organic cage pots by social self-sorting, R. L. Greenaway et al, Angew. Chem. Int. Ed., 2019, 131, 16421
[6] Machine learning for organic cage property prediction, L. Turkani et al, Chem. Mater., 2019, 31, 714
[7] Computational discovery of molecular C60 encapsulants with an evolutionary algorithms, M. Miklitz et al, Commun. Chem., 2020, 3, 10
Bio: Becky is a Royal Society University Research Fellow in the Department of Chemistry. After graduating with an MChem in Chemistry with Study in Industry from the University of Sheffield, Becky carried out her DPhil on palladium-catalysed cascade cyclisations under the supervision of Prof. Ed Anderson at the University of Oxford. She then worked with Prof. Andy Cooper FRS as a postdoctoral research associate at the University of Liverpool, where her research interests expanded to include the synthesis of porous organic cages leading to the realisation of the first molecular porous liquids in 2015. Her interests also include the use of high-throughput automation in the synthesis of molecular organic materials and supramolecular assemblies. Becky was awarded a Royal Society University Research Fellowship in 2019 on “Molecular Assembly in Porous Liquids”, allowing her to establish an independent research career in the Department of Chemistry at the University of Liverpool, before moving to Imperial in May 2020.