
- This event has passed.
10/11/2021 – AI3SD Autumn Seminar V: Quantum Machine Learning
10th November 2021 @ 1:00 pm - 3:45 pm
Free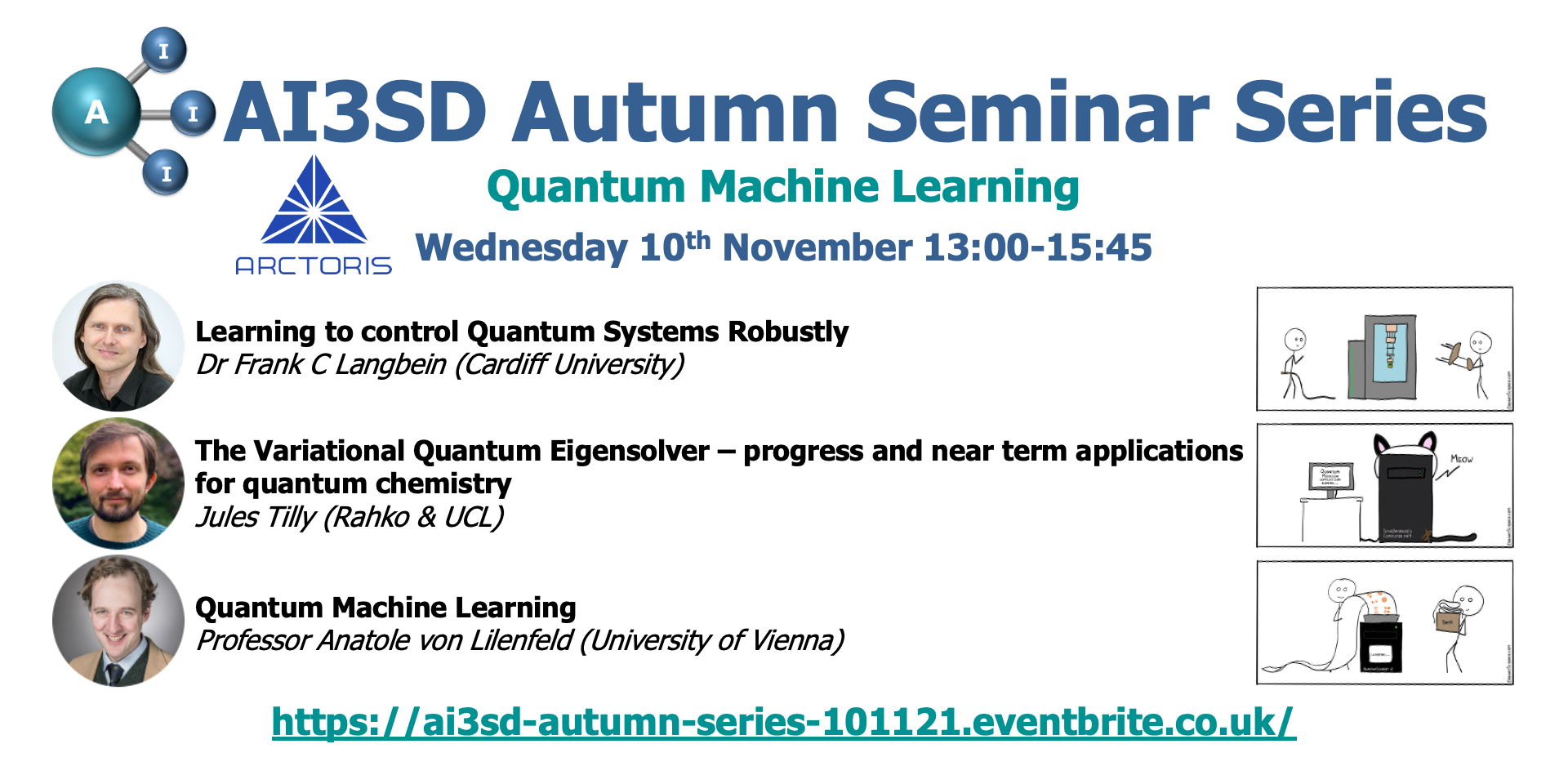
Eventbrite Link: https://ai3sd-autumn-series-101121.eventbrite.co.uk
Description:
This seminar forms part of the AI3SD Online Seminar Series that will run across the autumn (from October 2021 to December 2021). This seminar will be run via zoom, when you register on Eventbrite you will receive a zoom registration email alongside your standard Eventbrite registration email. Where speakers have given permission to be recorded, their talks will be made available on our AI3SD YouTube Channel. The theme for this seminar is Quantum Machine Learning.
Agenda
- 13:00-13:45: Learning to Control Quantum Systems Robustly –Ā Dr Frank C Langbein (Cardiff University)
- 13:45-14:00: Coffee Break
- 14:00-14:45: The Variational Quantum Eigensolver – progress and near term applications for quantum chemistry – Jules Tilly (Rahko & UCL)
- 14:45-15:00: Coffee Break
- 15:00-15:45: Quantum Machine Learning – Professor Anatole von Lilenfeld (University of Vienna)
Abstracts & Speaker Bios
- Learning to Control Quantum Systems Robustly – Dr Frank C Langbein: Quantum control provides methods to steer the dynamics of quantum systems. The robustness of such controls, in addition to high fidelity, is important for practical applications due to the presence of uncertainties arising from limited knowledge about system and control Hamiltonians, initial state preparation errors, and interactions with the environment leading to decoherence. We introduce a novel robustness measure based on the Wasserstein distance, and discuss structured singular value analysis and log-sensitivity approaches from classical robust control. This is employed to analyse the robustness of controllers found by reinforcement learning and gradient-based optimisation algorithms. Some, not all, high-fidelity controllers are also robust and controllers found by reinforcement learning appear less affected by noise than those found by gradient-based optimisation. We briefly discuss applications in information transfer in spin networks and magnetic resonance spectroscopy.
Bio: I am a senior lecturer inĀ Computer ScienceĀ atĀ Cardiff University, where I am a member of theĀ Visual Computing Research Section. I co-lead theĀ Qyber\blackĀ international research network in quantum control, which arose from the Quantum Technologies and Engineering research priority area at Cardiff University, and theĀ Healthcare Technologies Research Group at the School of Computer Science and Informatics. My research interests lie inĀ control,Ā machine learningĀ andĀ geometryĀ applied inĀ quantum technologies,Ā visual computing,Ā geometric modellingĀ andĀ healthcare. My teaching responsibilities cover individual and group projects and various programming techniques. See my websiteĀ Ex Tenebris ScientiaĀ for recent results and full details.
- The Variational Quantum Eigensolver – progress and near term applications for quantum chemistryĀ ā Jules Tilly: The Variational Quantum Eigensolver is among the most promising near term applications for quantum computing. It offers the possibility to model some wave functions accurately in polynomial time. Despite this, many hurdles and open questions remain. We will go through these questions, try discussing possible answers and the direction of research. After this we will discuss recent applications of the methods and integration to quantum chemistry methods such as CASSCF and experimentation on quantum computers.
Bio: Jules specialises in developing quantum machine learning methods for drug discovery with a focus on optimizing algorithm implementation on current / NISQ Quantum Computers. He is a Quantum Research Scientist at Rahko, and is currently completing his PhD at UCL under the supervision of Pr. J. Tennyson. Prior to this, Jules worked for 6+ years in financial services acting as regulatory and strategic advisor for global investment banks such Goldman Sachs, UBS and Citi Bank. He holds degrees in Mathematics, Quantum Physics, Law, Economics, Finance and Public Policy. - Quantum Machine LearningĀ – Professor Anatole von Lilenfeld:
Many of the most relevant observables of matter depend explicitly on atomistic and electronic details, rendering a first principles approach to computational materials design mandatory. Alas, even when using high-performance computers, brute force high-throughput screening of material candidates is beyond any capacity for all but the simplest systems and properties due to the combinatorial nature of compound space, i.e. all the possible combinations of compositional and structural degrees of freedom. Consequently, efficient exploration algorithms exploit implicit redundancies and correlations. I will discuss recently developed statistical learningĀ based approaches for interpolating quantum mechanical observables throughout compound space. Numerical results indicate promising performance in terms of efficiency, accuracy, scalability and transferability.
Bio: O. Anatole von Lilienfeld is a full university professor of computational materials discovery at the Faculty of Physics at the University of Vienna. Research in his laboratory deals with the development of improved methods for a first-principles-based understanding of chemical compound space using perturbation theory, machine learning, and high-performance computing. Previously, he was an associate and assistant professor at the University of Basel, Switzerland, and at the Free University of Brussels, Belgium. From 2007 to 2013, he worked for Argonne and Sandia National Laboratories after postdoctoral studies with Mark Tuckerman at New York University and at the Institute for Pure and Applied Mathematics at the University of California Los Angeles. In 2005, he was awarded a Ph.D. in computational chemistry from EPF Lausanne under the guidance of Ursula Rothlisberger. His diploma thesis work was done at ETH Zurich with Martin Quack and the University of Cambridge with Nicholas Handy. He studied chemistry at ETH Zurich, the Ecole de Chimie Polymers et Materiaux in Strasbourg, and the University of Leipzig. He serves as editor in chief of the IOP journal Machine Learning: Science and Technology and on the editorial board of Science Advances. He has been on the editorial board of Natureās Scientific Data from 2014 to 2019. He was the chair of the long IPAM āUCLA program āNavigating Chemical Compound Space for Materials and Bio Designāā which took place in 2011. He is the recipient of multiple awards including the Swiss National Science foundation postdoctoral grant (2005), Harry S. Truman postdoctoral fellowship (2007), Thomas Kuhn Paradigm Shift award (2013), Swiss National Science professor fellowship (2013), Odysseus grant from Flemish Science foundation (2016), ERC consolidator grant (2017), and Feynman Prize in Nanotechnology (2018).