Welcome & AI4SD Network Retrospective – Professor Jeremy Frey (University of Southampton)
The AI 4 Scientific Discovery Network, now rebranded as AI4SD has been running for nearly 4 years. This conference marks the end of our Network term and this talk will reflect on what AI4SD has achieved over the years, and where we are looking to go next.
Bio: Jeremy Frey is a Professor of Physical Chemistry and the head of the Computational Systems Chemistry Group at the University of Southampton. Before working at Southampton, he obtained his DPhil on experimental and theoretical aspects of van der Waals complexes in the Physical Chemistry Labs, Oxford University under the supervision of Professor Brian Howard, followed by a NATO/EPSRC fellowship at the Lawrence Berkeley Laboratory and University of California, with Professor Y. T. Lee. Jeremy’s experimental research probes molecular organisation in environments from single molecules in molecular beams to liquid interfaces using laser spectroscopy from the IR to soft X-rays. He investigates how e-Science infrastructure supports scientific research with an emphasis on the way digital infrastructure can enhance the intelligent creation, dissemination and analysis of scientific data.
Introducing the Future Blood Testing Network – Dr Weizi (Vicky) Li (University of Reading)
The Future Blood Testing Network+ is a new Network funded by EPSRC. We are aiming to build a multi-disciplinary community to develop digital health technologies for remote, rapid, affordable and inclusive monitoring and personalised analytics. This presentation will introduce our Network, detailing our plans for the next three years, in particular highlighting the funding calls and opportunities that will be relevant to the AI4SD Community.
Bio: Dr Weizi (Vicky) Li is an Associate Professor of Informatics and Digital Health, Deputy Director in Informatics Research Centre, Henley Business School, University of Reading. She is an interdisciplinary researcher focusing on using informatics, data science, machine learning, and digital information systems to solve real-world healthcare challenges. She is the academic lead of a large collaborative project of Improving the Quality of Healthcare through an Integrated Clinical Pathway Management Approach and Cloud based Digital Data Integration Platform, which was awarded ESRC O2RB Excellence in Impact Award in 2018 for her research impact on healthcare quality improvement. She is the academic lead of machine learning based decision support system for outpatient management which has successfully been implemented in Royal Berkshire NHS Foundation Trust and has received Research Engagement and Impact award in 2020. She has been PI on projects funded by ESRC, EPSRC, The Health Foundation, NHS and companies, working on data-driven decision support systems that use real-world data (under privacy preserving framework) from multiple sources including Electronic Patient Record in acute, community hospital and primary care settings, remote health monitoring and patient reported outcomes to develop novel technologies (including AI based methods) to support clinical and operational decision makings in patient pathway.
RSC CICAG “Who we are, what we do and what we are planning” – Dr Chris Swain (Cambridge MedChem Consulting)
The Royal Society of Chemistry Chemical Information and Computer Applications Group (CICAG) is one the the RSC’s member led interest groups. The storage, retrieval, analysis and preservation of chemical information and data are of critical importance for research, development and education in the chemical sciences. CICAG works to support users of chemical information by providing training workshops, conferences highlighting the latest research in the area, and to promote wider recognition of the importance of chemical information via the newsletter.
Bio: Chris Swain is Founder of Cambridge MedChem Consulting providing drug discovery expertise to a wide range of groups ranging from small academic groups, biotechs, though to small pharma and major international Pharma companies. Previously he was a Senior Director at Merck and was responsible for MedChem and Computational Chemistry activities, he was the Chemistry project director for the team that discovered the NK1 antagonist EMEND for which the team was awarded the RSC BMCS prize. He is also Chair of RSC CICAG and a committee member of the Royal Society of Chemistry Biological and Medicinal Chemistry Sector. He organises the Open Chemical Science workshops and is on the organising committees for the Cambridge MedChem and the Artificial Intelligence in Chemistry meetings. He also works with UCL to provide a computational chemistry for drug discovery course for MRes students.
Equality, Diversity & Inclusion in Networks: Developing your inclusive approach – Debra Fearnshaw (University of Nottingham)
This session will give delegates a framework in which to consider how they can develop an inclusive approach to their work, team and research. This talk will provide some examples of how to do this and inspire delegates to develop their own approaches.
Bio: Debra Fearnshaw completed a secondment to EPSRC in 2021 with another University of Nottingham secondee, Emma Hadfield-Hudson and together they developed a guide on Expectations for Equality, Diversity and Inclusion, in consultation with the research community. These aim to help support the Engineering and Physical Sciences community to achieve greater equality, diversity and inclusion in their research environment and give some practical examples of how ED&I has been implemented. Debra’s day job is Projects Manager within the Faculty of Engineering at University of Nottingham, managing 2 EPSRC research projects.
Translating innovations out of the lab and into the clinic: the importance of data curation, AI and ML? – Dr Jennifer Hiscock (University of Kent) & Thomas Allam (University of Southampton)
Our novel patented (European Patent Application No. 18743767.8, U.S. Patent Application No. 16/632,194), Supramolecular Self-associating Amphiphile (SSA) platform technology currently contains a library of ≈ 120 molecules (Figure 1), invented by J. Hiscock in 2016, has since been developed by an international, transdisciplinary team of ≈50 academic/industrial/governmental scientists, social scientists and clinicians. To date this molecular technology has been shown to:
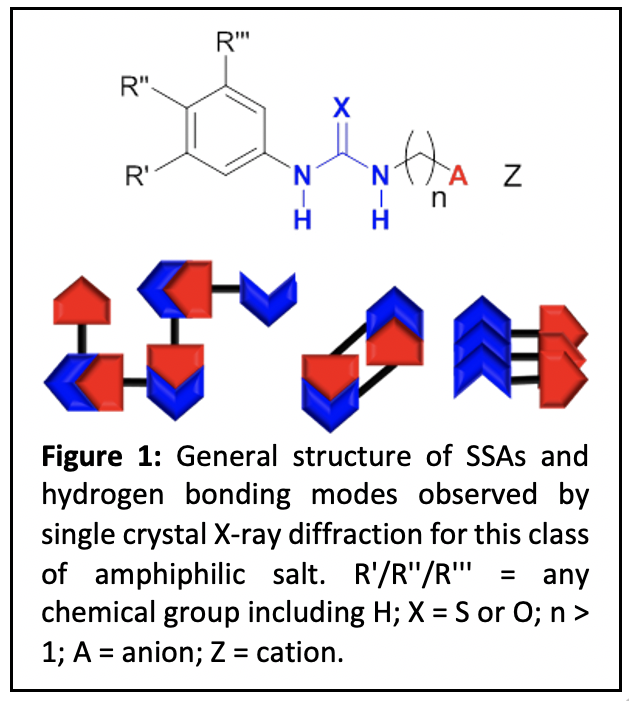
- act as broad-spectrum antimicrobials;1–6
- increase the efficacy of other antibiotic/antiseptic agents and anticancer agents against bacteria7 and ovarian cancer cells respectively;8
- selectively interact with phospholipid membranes of different compositions;9,10
- have the potential to act as drug delivery vehicles;11
- exhibits a drugable profile when administered by i.v. in vivo (unpublished data);
- and enable the production of novel flow battery electrolytes.12
However, this means the we not only create a lot of data, but that these multiple outputs exist in multiple forms, come from all over the world and are underutilised when designing the next project steps. Here we will attempt to introduce you to our approach in solving these problems.
References
(1) Chem. Commun., 2021, 57, 11839 – 11842; (2) Supramol. Chem., 2020, 32, 414–424; (3) J. Mater. Chem. B, 2020, 8, 4694–4700; (4) ChemMedChem, 2020, 15, 2193–2205; (5) Supramol. Chem., 2020, 32, 414–424; (6) Chem. Commun., 2019, 55, 95–98; (7) RSC Advances, 2021, 11, 9550 – 9556; (8) RSC Advances, 2021, 11, 14213 – 14217; (9) Chem. Commun., 2020, 56, 4015–4018; (10) Chem. Commun., 2020, 56, 11665–11668; (11) Molecules, 2020 , 25:18, 4126-4141; (12) Chem. Commun., 2020, 56, 11815-11818.
Bio: Dr Jennifer Hiscock obtained her PhD from the University of Southampton (UK) under the supervision of Prof. Philip A. Gale in 2010 studying supramolecular host:guest chemistry. She continued her post-doctoral research between this group and Dstl (Porton Down – UK) until 2015 when she moved to the University of Kent (UK) as the Caldin research fellow. In 2016 she was awarded a permanent lectureship position at that same institution, which has since been followed by her promotion to Reader in Supramolecular Chemistry and Director of Innovation and Enterprise for the School of Physical Sciences in 2019. In 2020 she was awarded a UKRI Future Leaders Fellowship, developing novel cell surface active therapeutics and drug adjuvants and in 2021 her efforts were recognised through the award of the consolidator prize for research by the University of Kent. Her research currently focuses on a transdisciplinary approach to applying supramolecular chemistry to solve real-world problems.
AI and multi-omics discovery science: A case study in understanding ageing at a systems level – Dr Janna Hastings (EFPL/UNIL & UCL)
Metabolism is central to all processes of life and the metabolome — large-scale measurement of the quantities of small molecular entities in cells and tissues — gives a readout of cellular functioning at a point in time. Harnessing metabolomic information together with transcriptomic information about gene expression allows for multi-level insights into genetic dysregulation and its cellular effects. I will describe a multi-omics approach based on genome-scale modelling that is able to integrate the two levels and provide insights into the systems-level deregulation of cellular function due to ageing by transforming the cellular reaction space into a constraint-based linear optimisation problem. Metabolic models such as these and their interpretation depends on publicly available data about small molecular metabolites. Chemical ontologies provide structured classifications of chemical entities that can be used for navigation and filtering of chemical space including in metabolic models. ChEBI is a prominent example of a chemical ontology, widely used in life science contexts including to annotate metabolites in genome-scale models, and recent work has involved using deep learning to automatically extend the ChEBI classification to a wider range of metabolites thus enhancing the benefit of genome-scale models for ageing systems research. Finally, I will discuss the role of artificial intelligence technologies in systems-level -omics research more generally.
Bio: I am a computer scientist interested in developing artificial intelligence-based computational systems to support research across the biological and social sciences. I am particularly interested in the interface between data science, i.e. algorithms for deriving inferences and predictions based on structured and unstructured data, and knowledge science, i.e. research that amasses, integrates and harnesses what we already know and channels that back towards efforts to make novel discoveries, towards a genuinely cumulative discovery frontier. To this end I have actively contributed to research in computational knowledge representation and reasoning, to community-wide knowledge integration via building semantic standards, and to scientific discovery research using computational approaches across a range of domains.
Inference from Medical Images: Subspaces for Low Data Regimes – Professor Mahesan Niranjan (University of Southampton)
Unlike in the field of visual scene recognition, where tremendous advances have taken place due to the availability of very large datasets to train deep neural networks, inference from medical images is often hampered by the fact that only small amounts of data may be available. When working with very small dataset problems, of the order of a few hundred items of data, the power of deep learning may still be exploited by using a model pre-trained on natural images as a feature extractor and carrying out classic pattern recognition techniques in this feature space, the so-called few-shot learning problem. In regimes where the dimension of this feature space is comparable to or even larger than the number of items of data, dimensionality reduction is a necessity and is often achieved by principal component analysis or singular value decomposition (PCA/SVD). In this paper, noting the inappropriateness of using SVD for this setting we explore two alternatives based on non-negative matrix factorization (NMF) and discriminant analysis. Using 14 different datasets spanning 11 distinct disease types we demonstrate that at low dimensions, discriminant subspaces achieve significant improvements over SVD and the original feature space. We also show that at modest dimensions, NMF is a competitive alternative to SVD in this setting. Joint work with Jiahui Liu, Keqiang Fan and Xiaohao Cai
Bio: Mahesan Niranjan is a Professor of Electronics and Computer Science at Southampton. Prior to this, he worked at the University of Cambridge as a Lecturer in Information Engineering. And the University of Sheffield as a Professor of Computer Science, where he also served as Head of Computer Science and Dean of Engineering. Mahesan works in the area of machine learning, and his research interests are in the algorithmic and applied aspects of the subject. He has worked on a range of applications of machine learning and neural networks including speech and language processing, computer vision and computational finance. Currently, the major focus of his research is in computational biology. Alongside his duties at Southampton University, he also often travels to other international universities to present his research and teach intense short courses in Machine Learning.
Reinforcement Learning Methods – Dr Stephen Gow (University of Southampton)
Reinforcement learning is a machine learning paradigm in which an agent learns to make decisions to achieve a long-term goal. In the past five years, the previously somewhat niche method has seen substantially increased interest from within the chemistry community, driven by the need for a machine learning approach to problems of planning and sequential decision making and recent developments in harnessing the power of neural networks to make reinforcement learning achievable for large problems. This talk will introduce the theory that underpins reinforcement learning, review its applications in chemistry to date with a particular focus on the field of drug discovery and molecular design, and consider the future of this still-developing approach.
Bio: Stephen Gow arrived at the University of Southampton as a mathematics and statistics student in 2011, graduating in 2014 and completing a PhD in computational modelling in 2020. After a short spell as a Knowledge Transfer Partnership Associate in Machine Learning, Semantic Web & Voice Automation Technologies, he is now a researcher working on projects including the application of machine learning in chemistry and estimation of population characteristics of refugee populations.
Outlier Detection in Scientific Data – Dr Jo Grundy (University of Southampton)
A range of outlier detection methods are discussed and tested on synthetic data. The application of these methods to real world scientific data is discussed.
Bio: Dr Jo Grundy graduated from Exeter University with a BSc in Chemistry in 1996, qualified as a teacher with a PGCE from Durham University then taught at Varndean School for a couple of years in Brighton, before doing an MSc in 1999, then DPhil in Organometallic chemistry at Sussex University. Between 2004 and 2006 I worked with Prof. Mathey at UC Riverside, before coming back to teach at a school in Duisburg Germany. I returned to the UK and taught chemistry and maths to A level, at Farnborough Sixth Form, then the Isle of Wight College, Barton Peveril and Itchen College. In 2018, did a second MSc in Artificial Intelligence at Southampton University, then worked as a Teaching Fellow in Computer Science for a year, before starting in my present job as a Research Fellow in Machine Learning.
Cross-architecture tuning of quantum devices faster than human experts – Dr Natalia Ares (University of Oxford)
A concerning consequence of quantum device variability is that the tuning of each qubit in a quantum circuit constitutes a time-consuming non-trivial process that has to be independently performed for each device, requiring a deep understanding of the particular device to be tuned and “muscle memory”. I will show a machine-learning based approach that can tune quantum devices completely automatically, regard less of the device architecture and being agnostic to the material realisation. Our algorithm was able to tune double quantum dot devices defined in a Si FinFET, a Ge/Sicore/shell nanowire, and both SiGe and AlGaAs/GaAs heterostructures, successfully accommodating the different modes of gate operation and noise characteristics. We report tuning times as fast as 10 minutes starting from scratch – well over an order of magnitude faster than what would be achievable by a dedicated expert human operator. Just as AlphaZero showed that the achievements of AlphaGo could be extended to learning to win at different board games without needing to be reprogrammed for each, so our result shows that cross-architecture tuning of quantum devices can be achieved using machine learning.
Bio: Natalia Ares is an Associate Professor at the Department of Engineering Science, a Tutorial Fellow in New College and a Royal Society University Research Fellow. Her research focuses on quantum device control. She develops machine learning algorithms for the automation of quantum device measurement and optimisation. She also harnesses the capabilities of nanoscale devices to explore thermodynamics in the quantum realm. She completed her PhD thesis at Université Grenoble Alpes, France, and her undergraduates’ studies at Universidad de Buenos Aires, Argentina.
Jazz as a Social Machine – Dr Thomas Irvine (University of Southampton)
Jazz has always been a sociotechnical activity in which “no one knows everything but everyone knows something.” It shares this quality with what Web Scientists call a “social machine.” This talk takes a novel generative jazz algorithm, the Jazz Transformer (JT), as a springboard for critical reflections on the changing relations between computer science, musicology, and the Digital Humanities by placing AI jazzin the context of social machines. It offers a “reverse engineering” of the JT and its accompanying data set, the Weimar Jazz Database, as a way to explore how thinking about generative jazz AI can uncover hidden relations between Music Information Retrieval, traditional editorial musicology, and critical and postcolonial theory (e.g. Latour, Derrida, Foucault, and Sylvia Wynter). The result is a new perspective on AI jazz that frames it not only as a series of technical operations but also a social process in which musicians, critical scholars, and technologists can find each other, in knowledge and action, in a new kind of social machine.
Bio: Thomas Irvine is Associate Professor and Director of Undergraduate Programmes in Music, and an Alan Turing Fellow. Like many students and staff in our department and university I have an international background. I was born in Munich to American parents and grew up in Stony Brook, NY, USA. After studying viola at conservatoire (at the Shepherd School of Rice University and Indiana University Jacobs School of Music) I moved to Germany and played professionally, mostly in Early Music ensembles but also in symphony orchestras. I also taught for a year at the Frankfurt International School and worked as a manager for a large Early Music organisation. In 1999 I found my way to musicology and back to the US, studying performance practice and musicology at Cornell University, where I took my PhD in 2005. In 2002 I crossed the Atlantic again as a DAAD scholar at the University of Würzburg Institute of Musicology, where I stayed on as a postdoctoral fellow in 2005/06. I have lived and worked in Southampton since 2006. I am a Fellow of the Alan Turing Institute (the UK’s national institution for AI and data science), a Non-Executive Director of the Southampton Web Science Institute and currently serve as an external examiner at the Royal Academy of Music. I co-chair the American Musicological Society study group ‘Global East Asia.’ Outside of my teaching and research I am trying to learn Chinese and follow Southampton FC. Both can be challenging! I also sing a little.
Interpretable Machine Learning for Materials’ Design and Characterisation – Dr Keith Butler (STFC)
“Where is the knowledge we have lost in information?” T.S. Eliot, The Rock
Machine learning (ML) and artificial intelligence (AI) are the subjects of wildly differing opinions on utility and potential impact. Depending who we talk to ML is the solution to almost every human challenge, from open boarders to pandemic control, or presents an existential crises for the species. In materials science the polarisation is perhaps less extreme, but nonetheless pervasive, while the numbers of ML related works experiences an explosion one highly respected theoretical chemist recently pronounced “[a]t least 50% of the machine learning papers I see regarding electronic structure are junk”. A part of the issue that many detractors have with ML methods is related to their perception of the techniques as ‘black-box’ approaches, at the same time, the same lack of understanding limitations of the models leads to some of the more outlandish boosterism surrounding the subject. In this talk I will discuss, with examples from our work, how we can open up the black-box of ML methods, highlighting and understanding limitations, increasing trust in results, and potentially improving the methods themselves.
Bio: Keith Butler is as a senior data scientist working on materials science research in the SciML team at Rutherford Appleton Laboratory. SciML is a team in the Scientific Computing Division and we work with the large STFC facilities (Diamond, ISIS Neutron and Muon Source and Central Laser Facility for example) to use machine learning to push the boundaries of fundamental science.
AI and optimisation in Computational Chemistry – Dr Grant Hill (University of Sheffield)
Numerical methods of optimisation are vital in chemistry, ranging from finding the lowest energy of a molecule through to reactor design. This talk will discuss two examples of how we are using optimisation techniques to work towards automating the discovery of new materials and developing computational chemistry methodology. The first of these is an AI3SD funded project where we set out to develop new membranes for water desalination using artificial intelligence techniques. In the second, we will demonstrate how the basis sets used in quantum chemistry calculations can be optimised in an automated fashion.
Bio: J. Grant Hill is a Senior Lecturer in theoretical chemistry at the University of Sheffield. The Hill research group aims to answer fundamental questions at the molecular level by developing and applying theory and computational tools. A current emphasis is on the use of machine learning to improve quantum chemical approaches.
Discovery of Synthesisable Organic Materials – Stephen Bennett (Imperial College London)
The computational discovery of new materials with useful properties is currently hindered by the difficulty in transitioning from a computational prediction to synthetic realisation. Attempts at experimental validation are often time-consuming, expensive, and frequently, the key bottleneck of material discovery.[1] Porous organic cages (POCs) have been discovered as a possible alternative material for molecular separations, catalysis, and sensing applications.[2] For POCs, a priori property prediction is possible,[3] however, it can be time-consuming and computationally expensive to explore a large number of possible candidate molecules. Despite being able to predict materials with exceptional properties, it is often challenging to predict whether it is possible to synthetically realise a potential candidate compound. In the field of drug discovery, machine learning techniques have been able to readily distinguish between synthesisable and unsynthesisable molecules, accelerating the drug discovery process.[4] Incorporating a synthetic accessibility scoring function into the precursor selection process favoured less complex, synthetically accessible precursors; thus, bridging the gap between computational screening and experimental synthesis of POCs. Using data-driven synthetic accessibility scoring techniques and high-throughput experimentation, we developed a POC screening workflow to accelerate discovery of experimentally realisable POC candidates, which we demonstrate using high-throughput, automated experimentation. Existing measures of synthetic accessibility are often tailored towards predicting synthesisable drug-like molecules, whose synthetic requirements often do not align with those of materials discovery programs. By redefining synthetic accessibility as a classification problem, we were able to develop an alternative model able to predict synthesisable materials precursors.[5] Biasing towards easy-to-synthesise precursors facilitated the synthesis of several precursors predicted to form shape-persistent POCs. Using these novel precursors, we were able to construct a precursor library able to be combined using automation, enabling the accelerated discovery of POCs and the construction of an experimentally derived POC reaction dataset. Using this dataset, we aim to develop a model able to predict POC formation, a question challenging to address using conventional computational methods. [1] Szczypiński, F. T.; Bennett, S.; Jelfs, K. E. Can We Predict Materials That Can Be Synthesised? Chem. Sci. 2021, 12 (3), 830–840. [2] Hasell, T.; Cooper, A. I. Porous Organic Cages: Soluble, Modular and Molecular Pores. Nat Rev Mater 2016, 1 (9), 16053. [3] Greenaway, R. L.; Jelfs, K. E. High‐Throughput Approaches for the Discovery of Supramolecular Organic Cages. ChemPlusChem 2020, 85 (8), 1813–1823. [4] C. W. Coley, L. Rogers, W. H. Green and K. F. Jensen, SCScore: Synthetic Complexity Learned from a Reaction Corpus, J. Chem. Inf. Model., 2018, 58, 252–261 [5] Bennett, S., Szczypiński, F.T., Turcani, L., Briggs, M.E., Greenaway, R.L., and Jelfs, K.E. (2021) Materials Precursor Score: Modeling Chemists’ Intuition for the Synthetic Accessibility of Porous Organic Cage Precursors. J. Chem. Inf. Model., 61 (9), 4342–4356.
Bio: Steven Bennett obtained his Master’s degree in Chemistry in 2018 from University College London (UCL). In October 2018, he joined the Jelfs Group at Imperial College London to work on the computational discovery of synthetically accessible functional materials, specifically porous organic cages. This work is supported by a PhD studentship from the Leverhulme Trust via the Leverhulme Centre for Functional Materials, which aims to develop his interest in sustainable, advanced material development.
Automated Rational Design of Metal-Organic Polyhedra – Dr Alexander Kondinski (University of Cambridge)
Metal-organic polyhedra (MOPs) are hybrid organic-inorganic nanomolecules, whose rational design depends on harmonious consideration of chemical complementarity and spatial compatibility between two or more types of chemical building units (CBUs). In this work, we apply knowledge engineering technology to automate the derivation of MOP formulations based on existing knowledge. For this purpose we have: i) curated relevant MOP and CBU data; ii) developed an assembly model concept that embeds rules in the MOP construction; iii) developed an OntoMOPs ontology that defines MOPs and their key properties; iv) software tools that populate the knowledge graph; and v) algorithm that using information from the knowledge graph derive a list of new constructible MOPs. Our result provides rapid and automated instantiation of MOPs in the knowledge graph, unveils the immediate chemical space of known MOPs, and sheds light on new MOP targets for future investigations.
Bio: Aleksandar Kondinski is a Feodor Lynen Fellow at the University of Cambridge. As a member of the CoMo Group (Prof. Markus Kraft), he is developing knowledge engineering tools that emulate the decision-making process of the inorganic chemist. Aleksandar studied chemistry at Jacobs University Bremen. During his graduate studies at the same university, he worked at the interface of experimental and theoretical polyoxometalate chemistry. Following his graduation in 2016, he has completed two postdoctoral fellowships at RWTH Aachen and KU Leuven dealing with magnetic and biofunctional inorganics respectively.
Interpreting Opacity: understanding gaps in our explanations of artificial neural networks – Dr Will McNeill (University of Southampton)
We know everything that goes on within artificial neural networks. We tend to know of all the data such systems have been trained on. And designers will be aware of the various design decisions, training algorithms and techniques that went into their construction, too. At the same time, leading AI designers tell us that their systems are in some sense uninterpretable, inexplicable or opaque. That’s puzzling. Drawing on discussions in the philosophy of neuroscience and science more generally, I will make use of this puzzle to try to advance our understanding of what explanations we lack with respect to ANNS; hence the nature and scope of explanation. The puzzle helps us to distinguish different phenomena in need of explanation, and some limits to the mechanistic explanatory strategies so often helpfully employed in the cognitive neurosciences.
Bio: William has been a lecturer in Philosophy at the University of Southampton since 2016 and is part of the Philosophy of Language, Philosophy of Mind and Epistemology Research Group. Prior to this he lectured at Kings College London, the University of York and Cardiff University. His research interests are centered on the epistemology of perception, social cognition and inferential knowledge.
Event detection in single-molecule data – how to find molecular signatures without (too many) prior assumptions – Professor Tim Albrecht (University of Birmingham)
Data from single-molecule experiments, such as from current-time or conductance-distance spectroscopy or sensors, are often “noisy” and characterised by complex molecular behaviour. In some cases, extracting the physically relevant information may be based on supervised approaches, i.e. where labelled data are available for training. In other cases, such data are either not available or it may simply be undesirable to make a priori assumptions about the molecular characteristics, for example to prevent loss of information and expectation bias.[1,2] This may require unsupervised methods or alternative approaches that put an emphasis on “what is not background?”, rather than “what does an event look like?”. In my talk, I will discuss some of the approaches we have taken, including some based on image recognition networks (AlexNet, VGG16),[3,4] and show those can be used to extract not only physically meaningful characteristics, but also previously unknown molecular behaviour.
[1] M. Lemmer et al., “Unsupervised vector-based classification of single-molecule charge transport data”, Nat. Commun. 2016, 7, art. no. 12922
[2] T. Albrecht et al., “Deep learning for single-molecule science”, Nanotechnol. 2017, 28, 423001.
[3] A. Vladyka, T. Albrecht, “Unsupervised classification of single-molecule data with autoencoders and transfer learning”, Machin. Learn.: Sci. Technol. 2020, 1, 035013.
[4] C. Weaver et al., “Unsupervised Classification of Voltammetric Data with Image Recognition and Dimensionality Reduction” (in preparation)
Bio: Tim studied Chemistry at the University of Essen in Germany from 1995-2000. Following brief research visits at the European Joint Research Centre in Ispra in Italy and the University of California at Berkeley, Tim graduated with a Diploma in Chemistry (equivalent to a Masters degree) in early 2000. After graduating, Tim joined Peter Hildebrandt’s group at the Max-Planck Institute for Radiation Chemistry (now Bioinorganic Chemistry) in 2000. Tim worked on charge transfer processes in natural and artificial heme proteins on metal surfaces using SER(R)S, single-crystal electrochemistry and electrochemical STM (in Jens Ulstrup’s group at the Technical Institute of Denmark (DTU). He obtained his PhD from the Technical University (TU) of Berlin in 2003 and afterwards returned to Ulstrup’s group as a postdoctoral fellow. In 2006, he moved to London to take up a lecturer position in Interfacial and Analytical Sciences in the Chemistry Department at Imperial College, where he was made Senior Lecturer in 2011 and then Reader in 2014. In 2017, Tim joined the faculty in the School of Chemistry at Birmingham University as Chair of Physical Chemistry and has been the School of Chemistry’s Director of Research since 2018. He is the coordinator of the School’s Interest Group “Data and Machine Intelligence”.
Making sense of highly flexible molecular simulations: Where AI can help and where not – Dr Christof Jager (University of Nottingham)
With simulating the dynamic behaviour of ever bigger molecular systems for longer simulation time we simultaneously achieve more realistic timescales and gain much better insights into the physiological relevant time dependent behaviour of molecular systems, but also generate significantly more data and thus pose new challenges for filtering noise and analysing the simulation data. In simulation analysis and data dimensionality reduction we often rely on linear dependencies and behaviour within the simulated timespan. This generally is true for systems that show slow structural or conformational transitions over the simulated timespan. For example, proteins and enzyme simulations that undergo large scale conformational changes can adequately be analysed by methods of principle component analysis (PCA) and analysing and visualising low frequency normal modes. However, much more flexible molecular systems undergoing multiple and seemingly chaotic conformational changes are posing challenges for their analysis. Here, we need to advance our analysis toolbox. Moreover, it is important to understand the flexibility and linear behaviour of the simulated system before choosing the analysis methods. In this talk we present three very differently behaving molecular systems including an enzymatic activation process, a flexible self-assembling host guest system, and a highly flexible dataset of lipid molecules relevant for antibiotic resistance of mycobacterium tuberculosis. We will show how chaos theory can help to understand the flexibility patterns of the simulations, then present classical PCA based simulation analysis, before introducing the opportunities and challenges for unsupervised machine learning methodologies. The presented methods will concentrate on competitive learning methods (self-organizing maps) and density based clustering algorithms (DB scan) to analysis dominating and hidden structural features in seemingly chaotic simulation data.
Bio: Christof Jäger is an Assistant Professor at the University of Nottingham working on computational chemistry in the field of enzyme design, biotechnology, supramolecular chemistry, and catalysis. Following his PhD in supramolecular computational chemistry at the Friedrich-Alexander-Universität (FAU) Erlangen- Nürnberg in Germany in 2010 and postdoctoral research on the design of organic electronic devices he moved to Nottingham in 2014. Since 2015 he worked as a Marie Curie COFUND and Nottingham Advanced Research Fellow on computational strategies for predictive enzyme engineering, before being promoted to his current position in 2018.
Physically-inspired deep learning of electronic structure for the design of tailormade functional molecules – Dr Reinhard Maurer (University of Warwick)
Materials for electronic devices such as organic light emitting diodes need to have tailored optoelectronic properties and be synthetically viable. As devices are composed of organic thin films with different functionality, the optoelectronic properties of materials need to be designed in concert and the role of the interfaces between films must be understood. Computational high-throughput screening of molecular excited states can greatly facilitate this complex multi-objective design problem. I will present our recent work on deep machine learning models that are able to predict structure, molecular electronic structure, and excited states of organic molecules and metal-organic interfaces. Our models predict optical excitations, the fundamental gap, electron affinity and ionisation potential for large organic molecules of diverse composition. While the models are trained on first principles electronic structure data, the prediction process requires no recourse to computationally expensive ab initio calculations. The accuracy and transferability of the models can be assessed against photoemission spectroscopy data. I will further showcase how such models can be used in combination with generative machine learning to discover novel organic compound combinations that satisfy specific optoelectronic properties.
Dr. Reinhard J. Maurer is an Associate Professor in the Department of Chemistry at the University of Warwick. He obtained his PhD in Theoretical Chemistry at the Technical University Munich in 2014, and then worked as a Postdoctoral Associate at Yale University until 2017 when he joined the University of Warwick. His research focuses on the theory and simulation of molecular reactions at surfaces and of hybrid organic-inorganic interfaces. He leads a research group of 5 postdoctoral researchers and 11 PhD students. He holds a UKRI Future Leaders Fellowship to develop simulation methods for nonadiabatic dynamics at metal surfaces and has recently been awarded an ERC Starting grant to develop machine learning models of electronic structure for the simulation of photocatalytic processes.
Learning from less than perfect crystals – Dr James Cumby (University of Edinburgh)
Based on knowledge of the types and positions of atoms in a material, it is increasingly possible to predict physical properties through simulation or statistical learning. A significant challenge remains, however, in that the simulated properties of a material frequently do not match reality. In part, this is due to deviations from the ‘ideal’ structure within crystals such as defects or disorder. This talk will introduce an approach for representing crystal structures for machine learning that can be applied equally to ordered and defective materials, and introduce some recent examples of how we can bridge the order-disorder gap.
Bio: James Cumby is a Lecturer in Inorganic Chemistry at the University of Edinburgh. He completed his PhD in Materials Chemistry at the University of Birmingham in 2014. His work examines the complex electronic, ionic and magnetic behaviour in metal oxide-fluoride materials. He is particularly interested in combining experimental techniques with data-driven approaches to discover new functional materials.
The Crystal Isometry Principle – Dr Vitaliy Kurlin (University of Liverpool)
The strongest and most practical equivalence of periodic crystals is rigid motion or isometry preserving all inter-atomic distances. The Crystal Isometry Principle (CRISP) says [1] that all real non-equivalent crystals should have non-isometric structures of atomic centres without chemical labels. If one atom is replaced by another one, distances to neighbouring atoms are inevitably perturbed, which can be detected by recent geometric invariants independent of any thresholds. More than 200 million pairwise comparisons of all periodic crystals with full geometric data from the Cambridge Structural Database (CSD) over two days on a modest desktop found five pairs of suspicious entries with different compositions but identical geometries [1, section 7]. For instance, all geometric parameters of HIFCAB and JEPLIA are identical to the last decimal place, but one atom of Cadmium is replaced by Manganese. With the help of the Cambridge Crystallographic Data Centre, all journals that published the underlying papers started investigations into data integrity. These experiments confirm that all periodic crystals (without restricting them to any chemical composition) live in a common Crystal Isometry Space (CRISP) parameterised by complete invariants. For example, diamond and graphite consisting of identical carbon atoms occupy in this CRISP space different positions given by unique geographic-style coordinates and a well-defined distance. In the same way, Mendeleev put all chemical elements (despite their obvious differences) into a single periodic table parameterised by two discrete coordinates: the period and group number. The new invariant coordinates extend Mendeleev’s table to the continuous space CRISP containing all existing and not yet discovered periodic crystals. [1] Widdowson, Mosca, Pulido, Kurlin, Cooper. Average Minimum Distances. MATCH Commun. Math. Comput. Chem. 87, 529-559 (2022), kurlin.org/projects/periodic-geometry-topology/AMD.pdf
Bio: Dr Vitaliy Kurlin is a Reader working in the Materials Innovation Factory at Liverpool since 2016. He completed a PhD in Geometry and Topology at Moscow State University in 2003 and held a Marie Curie International Incoming Fellowship in 2005-2007. Since 2018 he leads the Liverpool team of four co-Is in the £3.5M EPSRC grant `Application-driven Topological Data Analysis’ with Oxford. Since 2021 he is the Royal Academy of Engineering industry fellow at the Cambridge Crystallographic Data Centre. His Data Science group includes more than 10 researchers developing the new area of Periodic Geometry for applications in Crystallography.
AI Insights from Billions of Dollars of Ready-Cleaned Data – Dr Will Bowers (Dotmatics)
Two of the greatest pain points in Artificial Intelligence (AI)-assisted research workflows are data quantity and data organisation. Estimates place 60-80% of time in data science workflows is simply cleaning and arranging the data, dependent on researcher skill and the type of data. As AI relies on pattern recognition, the larger the dataset, the more likely the algorithm is to recognise a useful pattern. Due to organised unput via the Studies ELN and the underlying architecture of the database, extracting AI-ready data is made simple. We hold billions of dollars’ worth of data for our clients, and by working with each organisation to show them the untapped potential that existing projects already hold, then future research can be designed with these methodologies in mind to further boost research turnover and outcomes.
Bio: Will Bowers is a Data Scientist for Dotmatics, but that’s only scraping the surface of what they do. As well as building out Dotmatics’ Platform AI capabilities, they are a part time writer, LGBTQ+ style icon, and emergent supervillain. They have studied at the University of Leicester, the University of Tulsa, Imperial College London, and the Institute of Cancer Research, before finding a home as a founding member of Dotmatics’ recent Science and Technology Specialism Team.
Open Access Publishing & Open Data – Alexander Whiteside (Digital Discovery RSC)
Alex Whiteside provides an introduction to the Royal Society of Chemistry’s new open access journal Digital Discovery, guidance on publishing your work and some thoughts on open science and open data at the RSC.
Bio: Dr Alexander Whiteside is an Assistant Editor for the Royal Society of Chemistry, based near their offices in Cambridge, UK. As part of the RSC’s Open Access journals team, he supports the development and production of Digital Discovery and RSC Chemical Biology. Alex obtained a PhD in computational chemistry at Heriot-Watt University in 2012, and pursued postdoctoral research on computational materials chemistry at the University of Bath and the University of Cambridge before joining the RSC in 2016.
Sharing Data Science Solutions Across Domains via Patterns – Dr Sarah Callaghan (Patterns)
We are generating more data than we ever have before and are developing new and exciting ways to derive new insights from them. Sharing our research is a fundamental part of expanding humanity’s knowledge, as well as ensuring that researchers get credit for their work. In this talk I will introduce Patterns, the data science journal from Cell Press, along with wider discussions about the current state of AI and data science, and how to ensure that what we learn is shared and communicated effectively.
Bio: Sarah comes to Patterns from a 20-year career in creating, managing, and analyzing scientific data. Her research started as a combination of radio propagation engineering and meteorological modeling, then moved into data citation and publication, visualization, metadata, and data management for the environmental sciences. She was editor-in-chief of Data Science Journal for 4 years and has more than 100 publications. Her personal experience means she understands the frustrations that researchers can have with data. She believes that Patterns will bring together multidisciplinary groups to share knowledge and solutions to data-related problems, regardless of the original domain, for the benefit of humanity and the world.
Predictive Retrosynthesis in SciFindern – Dr Gary Gustafson (CAS)
The Retrosynthetic Planner in uncovers and prioritizes synthetic pathways to known and novel targets leveraging sophisticated algorithms mining the CAS REGISTRY® database. Each transformation is supported by literature evidence and possible alternatives steps are provided for review and substitution if desired.
Bio: Dr. Gustafson worked as a medicinal chemist for 25 years in biotech. Since joining CAS 3 years ago, he supports the sales team providing training on the CAS solutions and he also works with the product development team on the continuous improvement of the CAS suite of solutions.
Physical Sciences Data Infrastructure: shaping the physical sciences roadmap – Professor Simon Coles & Dr Nicola Knight (University of Southampton)
Digital technologies and computational resources are being utilised in scientific research and an increasing rate, however, the vast potential of these resources has yet to be realised. The physical sciences data infrastructure (PSDI) project aims to accelerate research in the physical sciences by providing a data infrastructure that brings together and builds upon the various data systems researchers currently use. This project is currently funded through the EPSRC digital research infrastructure funding to undertake a period of community engagement and requirements gathering.
Bios: Simon Coles is Professor of Structural Chemistry and Director of both the UK National Crystallography Service and the UK Physical Sciences Data-science Service. Simon obtained his BSc and PhD in structural systematics and molecular modelling at the University of Wales, Cardiff in 1992 and 1997 respectively. He held a Postdoctoral appointment with the Royal Institution, but based at the CCLRC Daresbury Laboratory, where he helped build the highly successful Small Molecule Single Crystal beamline, 9.8. In 1998 Simon moved to Southampton to establish a new laboratory and manage the National Crystallography Service. Simon transferred to Chemistry staff in July 2009, when he took over the role of Director of the National Crystallography Service. During the last 20 years he has been awarded a number of grants in the areas of Structural Chemistry, Information Management, eResearch and eLearning. He also became the Director of the UK Physical Sciences Data-science Service in 2019. Simon is an author on around 900 papers covering the areas of structural chemistry, support for chemical synthesis and chemical information and is one of the most prolific chemical crystallographers of all time (measured by number of contributions to the Cambridge Structural Database). He has served on many national and international facilities and professional society bodies and currently sits on the Editorial Boards of Crystallography Reviews and Supramolecular Chemistry.
Dr Nicola Knight is an Enterprise Research Fellow at the University of Southampton working on the Physical Sciences Data-Science Service (PSDS). She completed her Masters of Chemistry (MChem) at the University of Southampton previously before undertaking a PhD in Chemistry under the supervision of Professor Jeremy Frey. Her PhD focused on the interface between Chemistry and Computing with research in chemical modelling, remote experiments and the implementation of IoT technology in scientific research. Nicola’s current research interests are in the use of computing in scientific labs and notetaking with particular interest in IoT technologies and streamlining the research process.
Development of a full stack for digital R&D in chemistry and chemical process development – Professor Alexei Lapkin (University of Cambridge)
In order to enable seamless access to AI tools in research, it is necessary to transform how our laboratories are equipped. AI requires access to data, and it takes too long to gain access and to clean up datasets. Our experimental hardware is not ‘wired’ and is not accessible to algorithms. What is required is a development of data architecture that enables access to experimental and literature data both to a ‘human in the middle’ and fully algorithmic research tasks. In this talk I’ll present our joint effort with the group of Prof Markus Kraft to implement knowledge graph for ML workflow in chemical synthesis development, and the work @ iDMT centre in Cambridge on expanding this to a fully digital R&D in molecular sciences.
Bio: Alexei Lapkin graduated with an MChem from Novosibirsk State University, specialising in membrane gas separation. He then worked at Boreskov Institute of Catalysis prior to moving to the University of Bath where he was employed as a research officer, which allowed him to complete his PhD in the area of multiphase membrane catalysis.
AI standardization to enable digital development – Emelie Bratt (BSI)
Standardisation is often viewed hand in hand with legislation but there are significant differences in the purpose each serves. While both look to deliver a common level of safety and trust for industry as well as consumers standards are voluntary in nature. The role of standards is to respond to common problems identified across industry, through collaborative consensus base processes. It looks to produce guidance to bridge gaps in understanding and practices across sectors and nations. In the fast evolving landscape of AI, standards looks to build a solid foundation of trust through common languages and understandings, for industries to evolve through innovation without hindering design or commercial favouritism. BSI hosts an established committee with an ever growing membership including an extensive range of representation from academia, industry, to regulators that champion UK positions at European and international level. This presentation will explore the landscape of how standards are developed and the impact it will have on the AI community.
Bio: Emelie Bratt is a Lead Standards Development Manager at BSI (The British Standards Institution). For close to 10 years she has been heading up standards development efforts in a variety of subject areas with a focus on emerging technologies. Her current portfolio includes coordinating UK priorities as well as managing national committee contributions to international standards on artificial intelligence, quantum computing, trustworthy software and more. She has held committee manager roles for both UK and international technical committees. Over the years, Emelie has also gained working knowledge of the valuable connections between industry driven standards creation, regulatory development and compliance.
AI4SD & IoFT AI for Ethics Working Group: Introducing the Working Group & Our Methodologies: Moral IT Cards & Design Fiction – Dr Samantha Kanza (University of Southampton), Dr Naomi Jacobs (Lancaster University), Dr Peter Craigon (University of Nottingham)
Our Ethics for AI Working group was born out of a meeting held by the Internet of Food Things Network just before the pandemic to consider research challenges for developing a data trust system for the food sector. Our group came together through a shared interest in Ethics, and formed a working group to consider the ethical dimensions of digital collaboration in the food sector, such as the unintended consequence of AI. We have run several workshops and produced several papers as part of this work (1 published, 2 submitted awaiting review, 3 in progress). We used two key methodologies to explore these ethical issues: Design Fiction and the Moral IT Cards. Whilst our focus was on the food industry, we found these methods extremely useful in terms of considering different ethical aspects of technology and as such we believe they would be of great use to the wider scientific community. Our talk will introduce the working group and our activities, and explain how our methodologies can be put into practice for any Ethical Project.
Bios: Dr Samantha Kanza is an Senior Enterprise Fellow at the University of Southampton. She completed her MEng in Computer Science at the University of Southampton and then worked for BAE Systems Applied Intelligence for a year before returning to do an iPhD in Web Science (in Computer Science and Chemistry), which focused on Semantic Tagging of Scientific Documents and Electronic Lab Notebooks. She was awarded her PhD in April 2018. Samantha works in the interdisciplinary research area of applying computer science techniques to the scientific domain, specifically through the use of semantic web technologies and artificial intelligence. Her research includes looking at electronic lab notebooks and smart laboratories, to improve the digitization and knowledge management of the scientific record using semantic web technologies; and using IoT devices in the laboratory. She has also worked on a number of interdisciplinary Semantic Web projects in different domains, including agriculture, chemistry and the social sciences.
Naomi is Lecturer in Design Policy and Futures Thinking at Imagination Lancaster, the design-led interdisciplinary research group at Lancaster University. Her work crosses various disciplines including design, computer science, and social science. Naomi’s previous work has focused primarily on interaction; between individuals, communities, disciplines or sectors, and between people and technology and the media they consume. Naomi is particularly interested in the intersection between the digital and the physical, and how this impacts society on many axes. She has been part of a number of research projects looking at how technologies such as IoT and AI are being implemented and governed, and is interested in how design can be used to shape policy for new technologies. In particular, her current work focuses on issues such as trust, transparency, privacy and bias, using speculative design methods to explore implications of technology currently in development or proposed for the future.
Dr Peter Craigon is a Research Fellow in Ethics, Legislation and Engagement in Food and Agricultural Innovation within the Future Food Beacon of Excellence at the University of Nottingham. He is working with Professor Kate Millar and Professor Richard Hyde on developing tools to encourage ethical engagement and regulatory responsiveness for researchers working in food and agricultural innovation and beyond. He previously worked at the Horizon Digital Economy Research Institute at the University of Nottingham developing a tool to enable ethical design of IT based technologies and also at the University of Nottingham School of Veterinary Medicine and Science researching dog behaviour. Alongside this he completed a multidisciplinary PhD at the Horizon Centre for Doctoral Training also at the University of Nottingham exploring how social maps create knowledge about place.
Harnessing advanced algorithms to enable the automated optimisation of telescoped chemical reactions; Performance directed self-optimisation of bimetallic nanoparticle catalysts – Dr Thomas Chamberlain (University of Leeds)
The catalytic performance of nanoparticles is dependent on an extensive number of properties, reactions conditions and combinations thereof; however, very few methods employing multivariate closed-loop optimisation of nanoparticle catalysts have been reported to date. Here we demonstrate a machine learning-driven reactor platform for the performance directed synthesis of nanoparticle catalysts. Our experimental strategy uses an automated two-stage continuous flow reactor with decoupled residence times, allowing the precise synthesis of gold-silver nanoparticles (AuAgNP) with variable metal compositions, and subsequent performance analysis using a 4-nitrophenol reduction reaction. Quantification of the reaction conversion using inline UV-Vis spectroscopy enables the direct observation of the catalyst performance in real time, providing an efficient response for the performance directed synthesis of the most catalytically active nanoparticles. This approach paves the way for the rapid synthesis and optimisation of new nanoparticle catalysts, thereby streamlining the development of sustainable chemical processes. In terms of algorithm development, as many real-world optimisation problems consist of multiple conflicting objectives and constraints which can be composed of both continuous and discrete variables, we are addressing a significant issue. Given the inherent nature of continuous variables, i.e. they have a real value in the desired optimisation range of the variable, they are often easier to explore with a wider array of applicable optimisation techniques. Discrete variables, however, can take the form of integer values or categorical values (materials, reaction solvents). In many cases these optimisations can be expensive to evaluate in terms of time or monetary resources. It is therefore necessary to utilise algorithms that can efficiently guide the search towards the optimum set of conditions for a given problem to reduce costs. He will harness a mixed variable multi-objective Bayesian optimisation algorithm to tackle the problem of simultaneously exploring continuous and discrete variable in the same optimisation, which if successful with represent a step change in this AI field.
Bio: Dr Thomas Chamberlain comes from Matlock, in Derbyshire, and completed an MSci in Chemistry at the University of Nottingham in 2005. He was then awarded a University Interdisciplinary award to study a PhD with Professors Andrei Khlobystov and Neil Champness in Chemistry and Peter Beton in the School of Physics working on the synthesis of novel functional fullerene molecules and the subsequent formation of fullerene/carbon nanotube peapod structures. He received his PhD in 2009 and then joined the Nottingham Nanocarbon group as a post-doctoral research associate studying the use of supramolecular forces, such as van der Waals and H-bonding, to organise molecules in 1D and 2D arrays utilising carbon nanotubes as quasi 1D templates. During this position he established the application of carbon nanotubes as catalytic nanoreactors for the formation of novel molecular and nanostructured products and developed a wide variety of techniques to study the interactions of carbon and metal species at both atomic and bulk length scales. Dr Chamberlain moved to the University of Leeds in 2015, where he is currently an Associate Professor with an established independent research group within the Institute of Process Research and Development applying his understanding of nanomaterials to both fundamental and applied research challenges.
Internship Talk – High-throughput generation of chemical isomers for the development of molecular models of biocrude oils. Dr Francisco Martin-Martinez (Swansea University)
The identification of chemical species in complex fluid materials like biocrude oils, is problem that can be largely solved by a computational optimisation of a molecular design space to expand the limited experimental data. This is specially useful due to the intrinsic difficulties to characterise this bitumen-like materials. We used available experimental data to generate molecular models of any biocrude oil from different biomass sources (e.g., chitin, coffee grounds, algae), and we expand the molecular space beyond the initial characterisation to constitute structural datasets for the training of machine learning (ML) algorithms. We have developed an algorithm to automate the generation of structural isomers for any given molecule, as well as to perform high throughput DFT calculations and to provide the lowest energy molecular structures, the associated electron density, and the reactivity of each atom in the molecules, which is used to suggest biocrude upgrading models. The DFT results will constitute a series of ab-initio-refined models and expanded datasets for training ML algorithms in the future. These models can be used for further computational studies, like molecular dynamics simulations.
Bio: I am Senior Lecturer at the Chemistry Department of Swansea University, and research affiliate at the Massachusetts Institute of Technology (MIT). Before, I have been Research Scientist (2016-2019) and Postdoctoral Fellow (2014-2016) at MIT, and postdoctoral associate (2011-2013) at the Vrije Universiteit Brussel. I got my BSc and MSc in Chemical Engineering at the University of Granada (Spain), and my PhD in Theoretical and Computational Chemistry, from the same University. At Swansea, I direct the “Martin-Martinez Lab”, where I lead an interdisciplinary and multicultural research group focused on Nature-inspired computational materials. In addition to my work in academia, I am Chief Research Officer at Hyve Forum, a think tank on Circular Economy; advisor on strategic innovation at PODIUM Strategy and Marketing; co-instructor at Station1 (USA), a start-up on social-driven innovation and education; and scientific advisor at Sweetwater Energy, and 2050Materials.
Curated large inorganic datasets of Reconnected InChI, InChI and IUPAC name – Thomas Allam (University of Southampton)
Speaking about my experience with the Skills4Scientists Seminar series on e-chemistry, networking and careers and the AI4SD internship itself.
Bio: Thomas Allam – Postgraduate research master’s student at the University of Southampton with an interest in using cheminformatics and machine learning to solve chemical problems.
Internship Talk – Making music with automated processes and AI for the AI3SD Network Mubin Kazmi (University of Southampton)
Making the music for the AI3SD Network was an exciting and daunting project. In my talk I’ll walk through the process I used to find and create ideas for the music, while talking about the sounds and technologies I used to make a successful musical package.
Bio: Mubin Kazmi is producer and composer for film, games, and media marketing, and is currently studying at the University of Southampton.
Bayesian Optimisation in Chemistry – Rubaiyat Khondaker (University of Cambridge)
Recent work on the problem of optimising the yield of a chemical reaction has focused on Bayesian optimisation methods. We extend this work in several directions by: determining the effect on the performance of the optimiser of altering the acquisition function and batch size; testing the robustness of the optimiser by applying it to other existing reaction yield data sets; and applying the optimiser to the new problem domain of molecular power conversion efficiency in photovoltaic cells. The talk will give an overview of the results obtained from the project, including how this may guide future developments in this area.
Bio: Hello! I’m Rubaiyat and I was born in Bangladesh, moving to the UK when I was around 3 years old, where I’ve spent most of my life since. I’ve had a strong fascination with mathematics since my childhood and achieved varying degrees of successes at British and European Olympiads. I am currently pursuing a bachelor’s degree in mathematics it at the University of Cambridge. In addition, I enjoy programming, with my main language being python – recently, I gave a talk for the Cambridge mathematics society using animations I’d coded, which took about 5000 lines! Another one of my projects is a plugin I developed that allows me to import flashcards from text files to Anki, which I use on a regular basis. I’m looking forward to expanding my knowledge of programming to include machine learning techniques. Outside of maths and programming, I enjoy reading – I’ve started reading Frank Herbet’s Dune series, a captivating sci-fi classic. I also play the piano and clarinet – and of course, being into programming, I love playing video games!
The Summer School, but not as we know it! – Dr Martin Elliott (Kings College London)
How to engage with a summer school in the COVID 19 world.
Bio: Martin has a background of business and leadership experience gained within management and consultancy in chemistry related companies from start ups to multi nationals as well as having managed the EPSRC Directed Assembly Grand Challenge network. His commitment to the chemistry using industries has been recognised throughout his career and he has actively participated in various forums at both UK and European levels for various bodies including the Chemical Industries Association (CIA), the European Chemical Industry Council (CEFIC), the Royal Society of Chemistry (RSC) and the Society of Chemical Industry (SCI) where he has served as chair of the Science and Enterprise group. He also sat on the United Kingdom Chemical Stakeholders forum for three years. He particularly enjoys working in environments that bring industry and academia together.